You signed in with another tab or window. Reload to refresh your session.You signed out in another tab or window. Reload to refresh your session.You switched accounts on another tab or window. Reload to refresh your session.Dismiss alert
I think this formula should be written as:
After all, the discriminator get optimal when it outputs 0.5 for all input in the situation of original formula
The text was updated successfully, but these errors were encountered:
In the original paper, the content adversarial loss is:
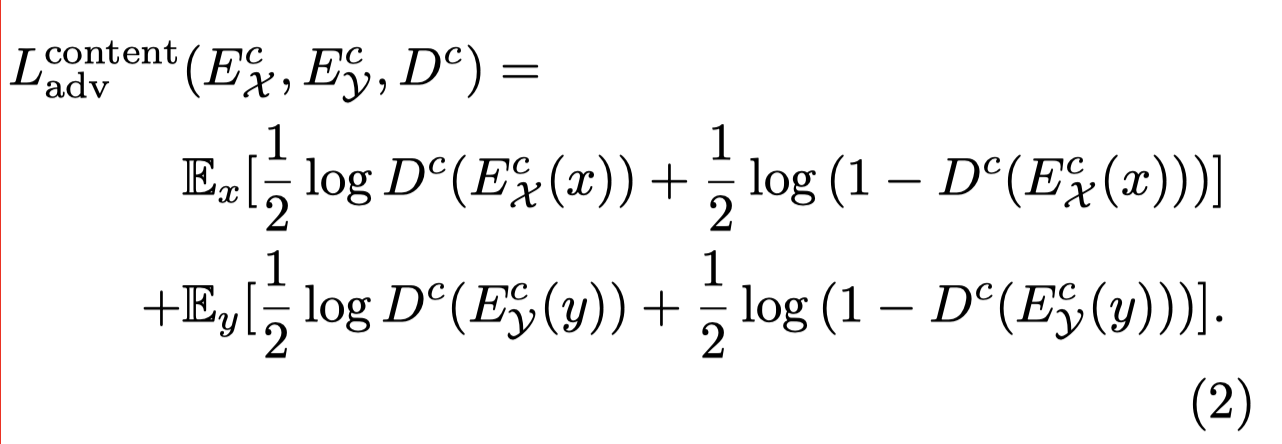
However, according to the code:
I think this formula should be written as:

After all, the discriminator get optimal when it outputs 0.5 for all input in the situation of original formula
The text was updated successfully, but these errors were encountered: