-
Notifications
You must be signed in to change notification settings - Fork 223
New issue
Have a question about this project? Sign up for a free GitHub account to open an issue and contact its maintainers and the community.
By clicking “Sign up for GitHub”, you agree to our terms of service and privacy statement. We’ll occasionally send you account related emails.
Already on GitHub? Sign in to your account
Overfitting? test AST on GTZAN #62
Comments
Dear Gong Yuan, Best regards, |
Hi Kelvin, First, you have 2000 * 30 clips and then crop each to 2 seconds? Why not crop it to 10s? Our model is trained on 10s and should well on that setting How did you instantiate your AST model? what is the When the input length is small, you will need to modify the specaug parameters. But I feel it should be better to crop to 10s. And what is your learning rate scheduler? Have you tried using a smaller learning rate? I'd suggest using your SOTA CNN hyper-parameters (e.g., batch size) and recipe (e.g., clip level eval), but just keep the input normalization the same as us and search the initial learning rate and learning rate scheduler. AST does converge faster (i.e., overfit faster) and needs a smaller learning rate. On ESC-50 (also 2000 samples), when the learning rate/learning rate scheduler is set correctly, the test performance always improves, see https://github.com/YuanGongND/ast/blob/master/egs/esc50/exp/test-esc50-f10-t10-pTrue-b48-lr1e-5/result.csv -Yuan |
FYI - in my own experiments, I use exactly the same hyparameters for CNN and AST except for the learning rate (AST uses 10 times smaller LR), see https://arxiv.org/pdf/2203.06760.pdf table 2. |
Dear Gong Yuan, For 2s, I initialized AST model using 192 t-dim. Yes, I will follow your suggestion to switch to 10s. About spec_aug, yes,I thought of that but did not try it due to my slow machine. Currently I am using 24 freqmask and 48 timemask, but I think you are right, I should using small timemask (maybe 12?) On LR and scheduler, I am try 1e-06 now. My scheduler is the same as you suggest in your paper --- Keep the original LR in the first 5 epoches (I am using 1e-04 on imagenet pretrained model, 1e-05 on imagenet + audioset pretrained model), after that, low down it by 0.85*previous LR in each epoch. On normalization, yes, I noticed the importance of normalization, I run "get_stats" to calculate my norm_mean and norm_std (GTZAN has norm_mean = -2.4281502, norm_std = 2.9490466) Actually I have a silly question on your normalization implementation: Yes, you are right, AST converged really fast, after one epoch, I got 80% accuracy as you see from my log: Thanks for your great work and especially thanks all for all the nice suggestions, I will report back my finding, Kelvin |
Dear Gong Yuan, Training ... Kelvin |
Thanks. From the learning curve, 1e-6 seems not to lead to performance over 90%. Trim to 2s and 10s makes the task difficulty different, I would still suggest to try 10s, get the norm stats for 10s audios (likely to be different with 2s), and search the learning rate.
I guess that would change the distribution and structure of the dataset. -Yuan |
Or, you can test your CNN baseline model with the 2s audios and compare the accuracy. In general, I think keeping the training/eval pipeline consistent makes a more fair comparison. |
Dear Gong Yuan, Thanks for any of your suggestion, |
I think it is worth trying a smaller learning rate. Add more time shifting as data augmentation might improve the performance a little bit ( Line 205 in 7b2fe70
You can also try Line 56 in 7b2fe70
What is your loss function? If it is a single-label classification problem (each clip has only one loss), you can also try CE loss instead of BCE. In general, I am wondering how you test your CNN model that achieves 92%? Is that possible to train AST with similar setting with it expect using a smaller learning rate and trimming audios to 10s? |
Dear Yuan, Thanks so much for your guidance, My loss function is BCEWithLogits, but previously I am using CE, I think they are identical in my 10-classes & single label music genre classification problem. To switch to BCEWithLogstic from CE, I copy your code to scalar label to lable vectors as following: label_indices = np.zeros(self.label_num) The way for me to achieve 92% using CNN is: So here when I work on AST solution, I am following the same logic, Have a nice day, |
Great to know thanks. I just thought CE is the more "correct" loss for single-label classification. Line 71 in 7b2fe70
Anyways I won't have time to check the detail. Good luck with your project. The reason I am suggesting using the same pipeline is in my experiments, I almost always find AST is slightly better than CNNs (my PSLA models), so I would be surprised otherwise. |
Dear Gong Yuan, One more question in my mind --- when I build 10s AST model, I also tried mixup, but seems it cause model hard to coverage. I noticed that you only use mixup in Audioset and SpeechCommand, how did you decide whether to apply mixup in your research work in general? I will make a fair comparing soon, |
Mixup can sometimes dramatically improve the performance (see my PSLA paper). I didn't use that with CE loss because I thought that might make the optimization harder, but I think it worth to have a try since you already move to BCE. |
Dear Gong Yuan, I forgot one thing --- with the above 10s segmentation-bases classifier, I tested the accuracy on 30s utterance level using majority voting approach (3 segments with 10s shift or more segment by a smaller shifting), accuracy is 91% :-) On CNN, I got 92%, but that is maybe due to a lot of tuning work. Anyway, it is really comparable, and I feel very happy for all the efforts because AST converge so quick. Thanks for your work! Have a nice day, |
Thanks, I learned a lot from your experience. There are certainly many things to tune, but one thing I noticed is you can actually input the entire 30s to AST without majority voting, to solve the memory issue, you can set I don't know much about music, PANNs (https://arxiv.org/pdf/1912.10211.pdf) report 91.5% on it, so your CNN is actually quite strong. |
Dear Gong Yuan, Then I realized that all my previous experiments were wrong maybe, because my signal is in 22050 HZ :-( So I start to down-sample my GTZAN data into 16KHZ, and try to repeat my experiment, but until now I still did not get the same performance yet. (what I got using 16HZ data is 88%, worse than the wrong experiment in 22KHZ in yesterday which is 91%) Still fine-tune LR for 16KHZ now. BTW, yesterday you mentioned that I can use: Thanks! |
Without AudioSet pretraining, higher sampling rate is better for music; Our AudioSet pretrained model is trained with 16kHz, so I guess it transfers better when the sampling rate is consistent, but I haven't done any experiment to verify this. When you use 10s audio input, have you tried to use our AudioSet norm stats?
Just use this scheduler, and change |
Hi Gong Yuan, The key factors lie in 2 pieces: 2> turn off mixup In all my experiments, I am using your imagenet + audioset pretrained model. I indeed applies audioset's norm stats in one of my experiments, my finding is that the performance is worse then using my own statistics. By looking at the value, I found that my norm_mean and norm_std are significantly different with audioset's, like the following: 2> my norm stats: Question: I have a guessing that mixup should be used very carefully, especially on how frequently it is used --- in your code, in 50% chance mixup will be called. Maybe I should try a smaller percentage because GTZAN only has 2000 utterances, say, to apply mix over 20% of data? Thanks for your hints on how to search for the optimal LR using ReduceLROnPlateau, I will do some experiments on it soon, because if I look back now over many of my endless running during the past two days, one lesson learn is to set a correct LR is crucial, and I actually search for the optimal LR manually which is too less efficient I guess :-(. Thanks so much, and have a nice day, |
Ask a simple question, how to calculate mean and std? |
Hi Dear Gong Yuan,
Thanks for your excellent work, I learnt a lot.
After understanding your code (partially), I test it on GTZAN using my own training framework (Just copy your ast_model.py)
GTZAN has 2000 music clips with roughly 30s duration per clip. My approach is to chop each clip into 2s segments to construct my data-set for training and testing (chop 70% clips to construct training set, remaining 30% clips as testing)
I tested both imagenet pretrained and imagenet + audioset pretraing model, accuracy looks comparable, but both reach a troublesome situation --- testing loss keep increasing while training loss decreasing. Looks like it is a typical overfitting.
Not sure if you have met the same situation when work on either ESC-50 or SpeechCommand? Let me paste my loss curves and accuracy here.
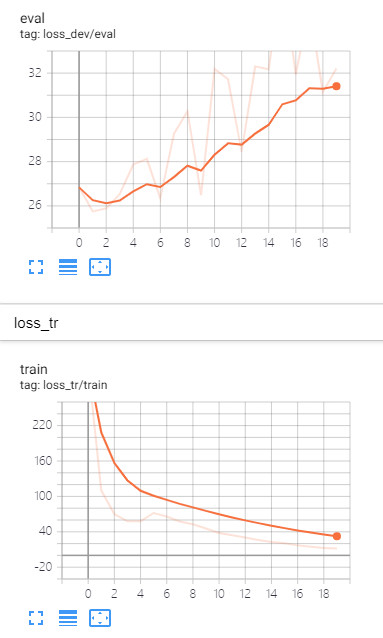
1> testing loss vs. training loss
2> testing accuracy:
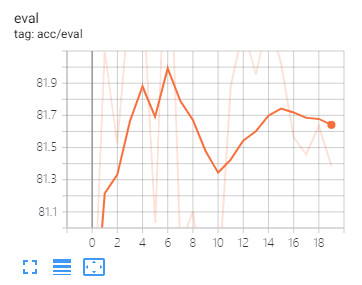
This accuracy looks competitive but not SOTA, because in another CNN approach, I can reach 92% accuracy easily on the same dataset.
My dataloader is very similar with yours, torchaudio to extract fbank in 128 dim, spec_augmentation, (0,0.5) normalization, etc.
I am using all same configuration as you suggested, batch size 48, lr (1e-04 or 1e-05), etc.
I don't worry its accuracy but more worry how can I make testing loss decreasing.
Thanks!
Kelvin
The text was updated successfully, but these errors were encountered: