forked from ultralytics/ultralytics
-
Notifications
You must be signed in to change notification settings - Fork 0
Commit
This commit does not belong to any branch on this repository, and may belong to a fork outside of the repository.
ultralytics 8.0.106
(ultralytics#2736)
Signed-off-by: dependabot[bot] <[email protected]> Co-authored-by: vyskocj <[email protected]> Co-authored-by: dependabot[bot] <49699333+dependabot[bot]@users.noreply.github.com> Co-authored-by: triple Mu <[email protected]> Co-authored-by: Ayush Chaurasia <[email protected]> Co-authored-by: pre-commit-ci[bot] <66853113+pre-commit-ci[bot]@users.noreply.github.com> Co-authored-by: Laughing <[email protected]>
- Loading branch information
1 parent
23fc506
commit 4db686a
Showing
41 changed files
with
1,159 additions
and
151 deletions.
There are no files selected for viewing
This file contains bidirectional Unicode text that may be interpreted or compiled differently than what appears below. To review, open the file in an editor that reveals hidden Unicode characters.
Learn more about bidirectional Unicode characters
This file contains bidirectional Unicode text that may be interpreted or compiled differently than what appears below. To review, open the file in an editor that reveals hidden Unicode characters.
Learn more about bidirectional Unicode characters
This file contains bidirectional Unicode text that may be interpreted or compiled differently than what appears below. To review, open the file in an editor that reveals hidden Unicode characters.
Learn more about bidirectional Unicode characters
This file contains bidirectional Unicode text that may be interpreted or compiled differently than what appears below. To review, open the file in an editor that reveals hidden Unicode characters.
Learn more about bidirectional Unicode characters
Original file line number | Diff line number | Diff line change |
---|---|---|
@@ -1,7 +1,78 @@ | ||
--- | ||
comments: true | ||
description: Learn about the Caltech-101 dataset, a collection of images for object recognition tasks in machine learning and computer vision algorithms. | ||
--- | ||
|
||
# 🚧 Page Under Construction ⚒ | ||
# Caltech-101 Dataset | ||
|
||
This page is currently under construction!️ 👷Please check back later for updates. 😃🔜 | ||
The [Caltech-101](https://data.caltech.edu/records/mzrjq-6wc02) dataset is a widely used dataset for object recognition tasks, containing around 9,000 images from 101 object categories. The categories were chosen to reflect a variety of real-world objects, and the images themselves were carefully selected and annotated to provide a challenging benchmark for object recognition algorithms. | ||
|
||
## Key Features | ||
|
||
- The Caltech-101 dataset comprises around 9,000 color images divided into 101 categories. | ||
- The categories encompass a wide variety of objects, including animals, vehicles, household items, and people. | ||
- The number of images per category varies, with about 40 to 800 images in each category. | ||
- Images are of variable sizes, with most images being medium resolution. | ||
- Caltech-101 is widely used for training and testing in the field of machine learning, particularly for object recognition tasks. | ||
|
||
## Dataset Structure | ||
|
||
Unlike many other datasets, the Caltech-101 dataset is not formally split into training and testing sets. Users typically create their own splits based on their specific needs. However, a common practice is to use a random subset of images for training (e.g., 30 images per category) and the remaining images for testing. | ||
|
||
## Applications | ||
|
||
The Caltech-101 dataset is extensively used for training and evaluating deep learning models in object recognition tasks, such as Convolutional Neural Networks (CNNs), Support Vector Machines (SVMs), and various other machine learning algorithms. Its wide variety of categories and high-quality images make it an excellent dataset for research and development in the field of machine learning and computer vision. | ||
|
||
## Usage | ||
|
||
To train a YOLO model on the Caltech-101 dataset for 100 epochs, you can use the following code snippets. For a comprehensive list of available arguments, refer to the model [Training](../../modes/train.md) page. | ||
|
||
!!! example "Train Example" | ||
|
||
=== "Python" | ||
|
||
```python | ||
from ultralytics import YOLO | ||
# Load a model | ||
model = YOLO('yolov8n-cls.pt') # load a pretrained model (recommended for training) | ||
# Train the model | ||
model.train(data='caltech101', epochs=100, imgsz=416) | ||
``` | ||
|
||
=== "CLI" | ||
|
||
```bash | ||
# Start training from a pretrained *.pt model | ||
yolo detect train data=caltech101 model=yolov8n-cls.pt epochs=100 imgsz=416 | ||
``` | ||
|
||
## Sample Images and Annotations | ||
|
||
The Caltech-101 dataset contains high-quality color images of various objects, providing a well-structured dataset for object recognition tasks. Here are some examples of images from the dataset: | ||
|
||
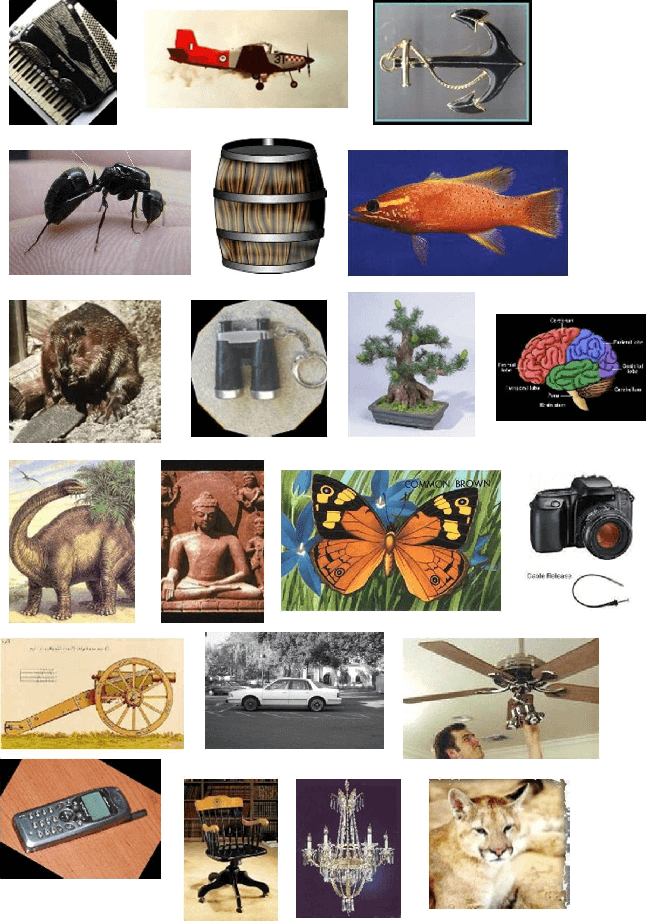 | ||
|
||
The example showcases the variety and complexity of the objects in the Caltech-101 dataset, emphasizing the significance of a diverse dataset for training robust object recognition models. | ||
|
||
## Citations and Acknowledgments | ||
|
||
If you use the Caltech-101 dataset in your research or development work, please cite the following paper: | ||
|
||
```bibtex | ||
@article{fei2007learning, | ||
title={Learning generative visual models from few training examples: An incremental Bayesian approach tested on 101 object categories}, | ||
author={Fei-Fei, Li and Fergus, Rob and Perona, Pietro}, | ||
journal={Computer vision and Image understanding}, | ||
volume={106}, | ||
number={ | ||
1}, | ||
pages={59--70}, | ||
year={2007}, | ||
publisher={Elsevier} | ||
} | ||
``` | ||
|
||
We would like to acknowledge Li Fei-Fei, Rob Fergus, and Pietro Perona for creating and maintaining the Caltech-101 dataset as a valuable resource for the machine learning and computer vision research community. For more information about the Caltech-101 dataset and its creators, visit the [Caltech-101 dataset website](https://data.caltech.edu/records/mzrjq-6wc02). |
This file contains bidirectional Unicode text that may be interpreted or compiled differently than what appears below. To review, open the file in an editor that reveals hidden Unicode characters.
Learn more about bidirectional Unicode characters
Original file line number | Diff line number | Diff line change |
---|---|---|
@@ -1,7 +1,73 @@ | ||
--- | ||
comments: true | ||
description: Learn about the Caltech-256 dataset, a broad collection of images used for object classification tasks in machine learning and computer vision algorithms. | ||
--- | ||
|
||
# 🚧 Page Under Construction ⚒ | ||
# Caltech-256 Dataset | ||
|
||
This page is currently under construction!️ 👷Please check back later for updates. 😃🔜 | ||
The [Caltech-256](https://data.caltech.edu/records/nyy15-4j048) dataset is an extensive collection of images used for object classification tasks. It contains around 30,000 images divided into 257 categories (256 object categories and 1 background category). The images are carefully curated and annotated to provide a challenging and diverse benchmark for object recognition algorithms. | ||
|
||
## Key Features | ||
|
||
- The Caltech-256 dataset comprises around 30,000 color images divided into 257 categories. | ||
- Each category contains a minimum of 80 images. | ||
- The categories encompass a wide variety of real-world objects, including animals, vehicles, household items, and people. | ||
- Images are of variable sizes and resolutions. | ||
- Caltech-256 is widely used for training and testing in the field of machine learning, particularly for object recognition tasks. | ||
|
||
## Dataset Structure | ||
|
||
Like Caltech-101, the Caltech-256 dataset does not have a formal split between training and testing sets. Users typically create their own splits according to their specific needs. A common practice is to use a random subset of images for training and the remaining images for testing. | ||
|
||
## Applications | ||
|
||
The Caltech-256 dataset is extensively used for training and evaluating deep learning models in object recognition tasks, such as Convolutional Neural Networks (CNNs), Support Vector Machines (SVMs), and various other machine learning algorithms. Its diverse set of categories and high-quality images make it an invaluable dataset for research and development in the field of machine learning and computer vision. | ||
|
||
## Usage | ||
|
||
To train a YOLO model on the Caltech-256 dataset for 100 epochs, you can use the following code snippets. For a comprehensive list of available arguments, refer to the model [Training](../../modes/train.md) page. | ||
|
||
!!! example "Train Example" | ||
|
||
=== "Python" | ||
|
||
```python | ||
from ultralytics import YOLO | ||
# Load a model | ||
model = YOLO('yolov8n-cls.pt') # load a pretrained model (recommended for training) | ||
# Train the model | ||
model.train(data='caltech256', epochs=100, imgsz=416) | ||
``` | ||
|
||
=== "CLI" | ||
|
||
```bash | ||
# Start training from a pretrained *.pt model | ||
yolo detect train data=caltech256 model=yolov8n-cls.pt epochs=100 imgsz=416 | ||
``` | ||
|
||
## Sample Images and Annotations | ||
|
||
The Caltech-256 dataset contains high-quality color images of various objects, providing a comprehensive dataset for object recognition tasks. Here are some examples of images from the dataset ([credit](https://ml4a.github.io/demos/tsne_viewer.html)): | ||
|
||
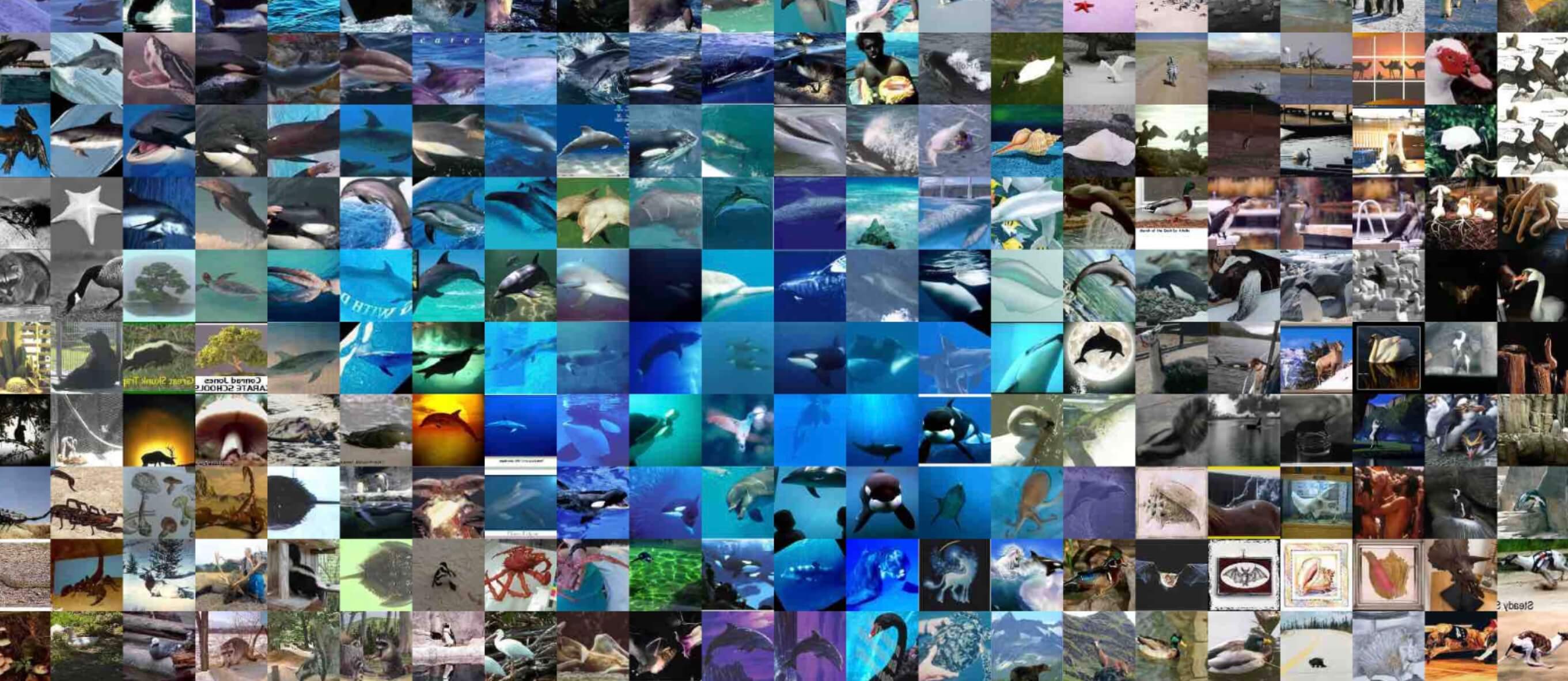 | ||
|
||
The example showcases the diversity and complexity of the objects in the Caltech-256 dataset, emphasizing the importance of a varied dataset for training robust object recognition models. | ||
|
||
## Citations and Acknowledgments | ||
|
||
If you use the Caltech-256 dataset in your research or development work, please cite the following paper: | ||
|
||
```bibtex | ||
@article{griffin2007caltech, | ||
title={Caltech-256 object category dataset}, | ||
author={Griffin, Gregory and Holub, Alex and Perona, Pietro}, | ||
year={2007} | ||
} | ||
``` | ||
|
||
We would like to acknowledge Gregory Griffin, Alex Holub, and Pietro Perona for creating and maintaining the Caltech-256 dataset as a valuable resource for the machine learning and computer vision research community. For more information about the | ||
|
||
Caltech-256 dataset and its creators, visit the [Caltech-256 dataset website](https://data.caltech.edu/records/nyy15-4j048). |
This file contains bidirectional Unicode text that may be interpreted or compiled differently than what appears below. To review, open the file in an editor that reveals hidden Unicode characters.
Learn more about bidirectional Unicode characters
Original file line number | Diff line number | Diff line change |
---|---|---|
@@ -1,7 +1,75 @@ | ||
--- | ||
comments: true | ||
description: Learn about the CIFAR-10 dataset, a collection of images that are commonly used to train machine learning and computer vision algorithms. | ||
--- | ||
|
||
# 🚧 Page Under Construction ⚒ | ||
# CIFAR-10 Dataset | ||
|
||
This page is currently under construction!️ 👷Please check back later for updates. 😃🔜 | ||
The [CIFAR-10](https://www.cs.toronto.edu/~kriz/cifar.html) (Canadian Institute For Advanced Research) dataset is a collection of images used widely for machine learning and computer vision algorithms. It was developed by researchers at the CIFAR institute and consists of 60,000 32x32 color images in 10 different classes. | ||
|
||
## Key Features | ||
|
||
- The CIFAR-10 dataset consists of 60,000 images, divided into 10 classes. | ||
- Each class contains 6,000 images, split into 5,000 for training and 1,000 for testing. | ||
- The images are colored and of size 32x32 pixels. | ||
- The 10 different classes represent airplanes, cars, birds, cats, deer, dogs, frogs, horses, ships, and trucks. | ||
- CIFAR-10 is commonly used for training and testing in the field of machine learning and computer vision. | ||
|
||
## Dataset Structure | ||
|
||
The CIFAR-10 dataset is split into two subsets: | ||
|
||
1. **Training Set**: This subset contains 50,000 images used for training machine learning models. | ||
2. **Testing Set**: This subset consists of 10,000 images used for testing and benchmarking the trained models. | ||
|
||
## Applications | ||
|
||
The CIFAR-10 dataset is widely used for training and evaluating deep learning models in image classification tasks, such as Convolutional Neural Networks (CNNs), Support Vector Machines (SVMs), and various other machine learning algorithms. The diversity of the dataset in terms of classes and the presence of color images make it a well-rounded dataset for research and development in the field of machine learning and computer vision. | ||
|
||
## Usage | ||
|
||
To train a YOLO model on the CIFAR-10 dataset for 100 epochs with an image size of 32x32, you can use the following code snippets. For a comprehensive list of available arguments, refer to the model [Training](../../modes/train.md) page. | ||
|
||
!!! example "Train Example" | ||
|
||
=== "Python" | ||
|
||
```python | ||
from ultralytics import YOLO | ||
# Load a model | ||
model = YOLO('yolov8n-cls.pt') # load a pretrained model (recommended for training) | ||
# Train the model | ||
model.train(data='cifar10', epochs=100, imgsz=32) | ||
``` | ||
|
||
=== "CLI" | ||
|
||
```bash | ||
# Start training from a pretrained *.pt model | ||
yolo detect train data=cifar10 model=yolov8n-cls.pt epochs=100 imgsz=32 | ||
``` | ||
|
||
## Sample Images and Annotations | ||
|
||
The CIFAR-10 dataset contains color images of various objects, providing a well-structured dataset for image classification tasks. Here are some examples of images from the dataset: | ||
|
||
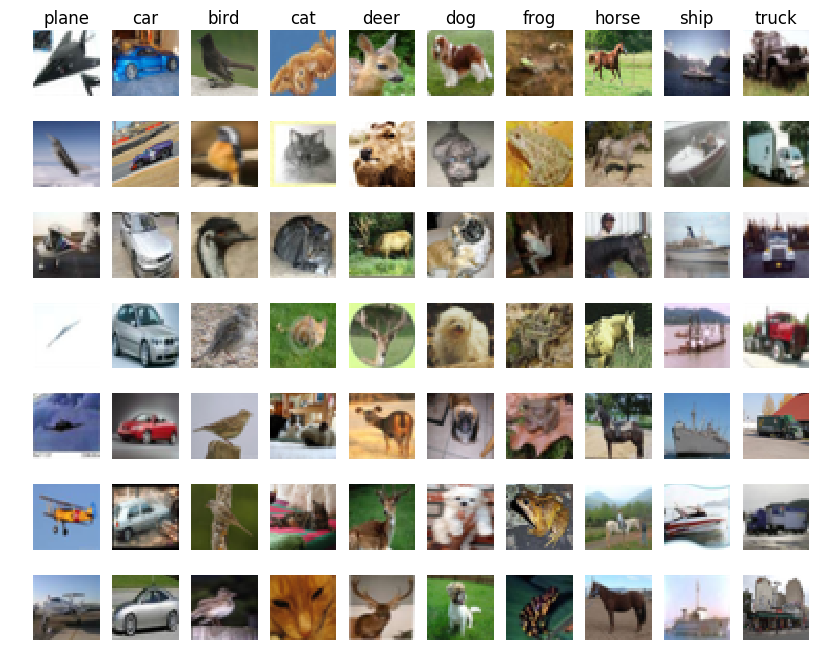 | ||
|
||
The example showcases the variety and complexity of the objects in the CIFAR-10 dataset, highlighting the importance of a diverse dataset for training robust image classification models. | ||
|
||
## Citations and Acknowledgments | ||
|
||
If you use the CIFAR-10 dataset in your research or development work, please cite the following paper: | ||
|
||
```bibtex | ||
@TECHREPORT{Krizhevsky09learningmultiple, | ||
author = {Alex Krizhevsky}, | ||
title = {Learning multiple layers of features from tiny images}, | ||
institution = {}, | ||
year = {2009} | ||
} | ||
``` | ||
|
||
We would like to acknowledge Alex Krizhevsky for creating and maintaining the CIFAR-10 dataset as a valuable resource for the machine learning and computer vision research community. For more information about the CIFAR-10 dataset and its creator, visit the [CIFAR-10 dataset website](https://www.cs.toronto.edu/~kriz/cifar.html). |
Oops, something went wrong.